Synthetic Neurocomputers for Cognitive Information Processing
Background
We all know Artificial intelligence (AI) , which is based on deep-neural-network models. And this AI has transformed the state-of-the-art in many domains, such as speech, visual-object recognitions, drug discovery, etc. In fact, current AI has already outperformed humans in some complicated tasks, marked by these two landmark demonstrations, Google’s AlphaGo defeated human champions in the Go game and IBM’s Watson won the quiz show “Jeopardy”.
However, despite the impressive progress, the capability of current AI technologies is still far from that of human brains. One key bottleneck is that even the most advanced silicon-based semiconductor devices perform information processing with orders of magnitude lower energy efficiency compared to neural cells. Moreover, the information processing and learning algorithms in current deep-neural-network models are only bio-inspired but not bio-identical. Current deep-learning models are only based on our limited knowledge of the inner working principles of neurons and synapses (so we have all this artificial neuron and synapses), also, they are based on the restricted functionality of conventional semiconductor devices and interconnects. However, in biological neural networks, neurons can fire asynchronously in parallel, with information carried by the signal amplitude, firing frequency, or firing mode, which leads to more complex and energy-efficient learning and computing.
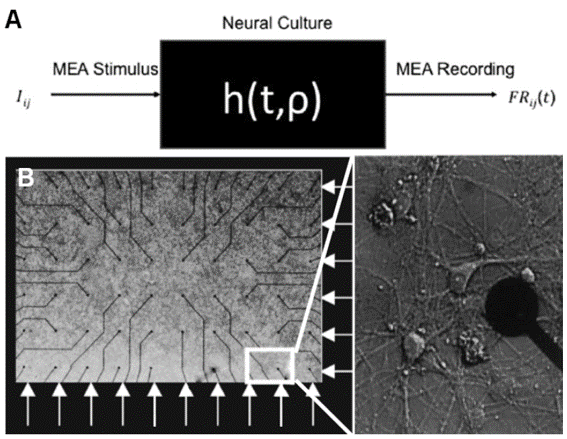
One natural approach to overcome the above-mentioned limitations is directly recruiting biological neurons into the biosynthetic computers, which harness the processing power of neural cells and their interconnected networks to perform cognitive computations.
In prior work, as early as 2000s, neurons were cultured on top of microelectrode arrays (MEAs). Electrical signals were then sent back and forth between electrodes and neurons as simple global inputs and outputs. Based on the recorded global, network-level neuronal activities, these cultured neural networks can have a lot of potential applications as listed here. Despite these impressive proof-of-concept demonstrations, the format of these neurocomputers is like “black boxes” as illustrated here in the Figure. The specific details of connectivity, network topology, and activity within the cultured neural networks are completely unknown
Synthetic Neurocomputers
To uncover the black-boxes, we aim to create a neurocomputer platform, as here, which can further allow us open the black-box by extracting the computing and learning algorithms of biological neural networks. And we hope that by revealing the black-box, we can have the potential to design “neuromorphic” or “cytomorphic” computing architectures that do not exist today.
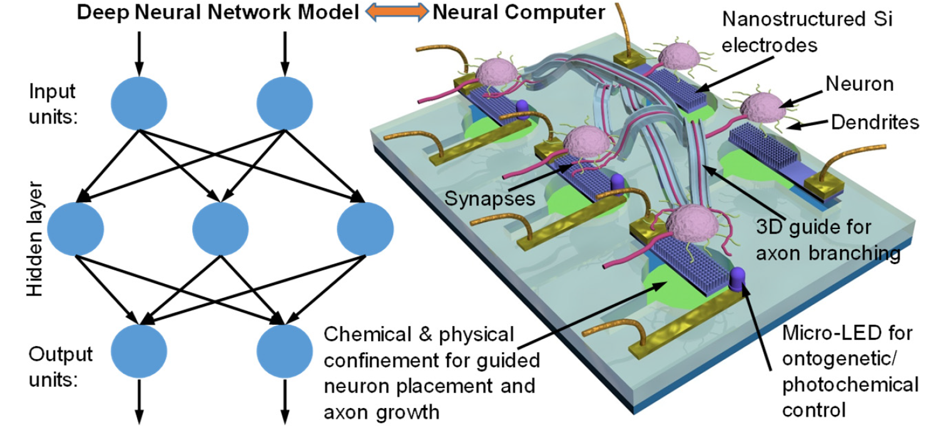
So how this platform will work? Specifically, the system will contain mainly 3 parts: the first is the complex but with well-defined topologies 3D guided cultured neural networks with controllable neuron cells growth at each nodes and axon branching across the 3D bridges. Meanwhile, neurons at each node are interfaced with u-LED devices, which are heterogeneously integrated through transfer-printing method. The reason why we want to use Optical signals as input is optical signals can offer unique advantages such as easily adjustable intensity as analog input, biocompatibility when combined with optogenetic, etc. We hope to investigate how this optogenetic process will affect the network output at different nodes, which will be captured by the third part of our system, our transferred nanostructured Si electrodes. The reasons that LEDs are the preferred light sources for neural stimulation are their small form factor and their adjustable wavelength.